Today organizations around the world are focusing on data to drive better results. They are willing to offer excellent services and look for developing various better products for their customers. It is a known fact that data can play a crucial part in helping companies reach their desired goals and objectives in this digitalized era. But can data alone i.e., the raw material is sufficient to generate insights in order to create value? In this article, we will understand how the data is well handled to achieve the expected business outcomes.
A comprehensive study on data’s growing importance in modern times
Digital transformation is required in the new normal. Most importantly, it isn’t achievable without a strong data management. Therefore, in this section we will look into an extensive study about data management, data value chain and its significance in the modern world. So, let’s start by understanding three important aspects of data management recommended by the data driven organisation mckinsey.
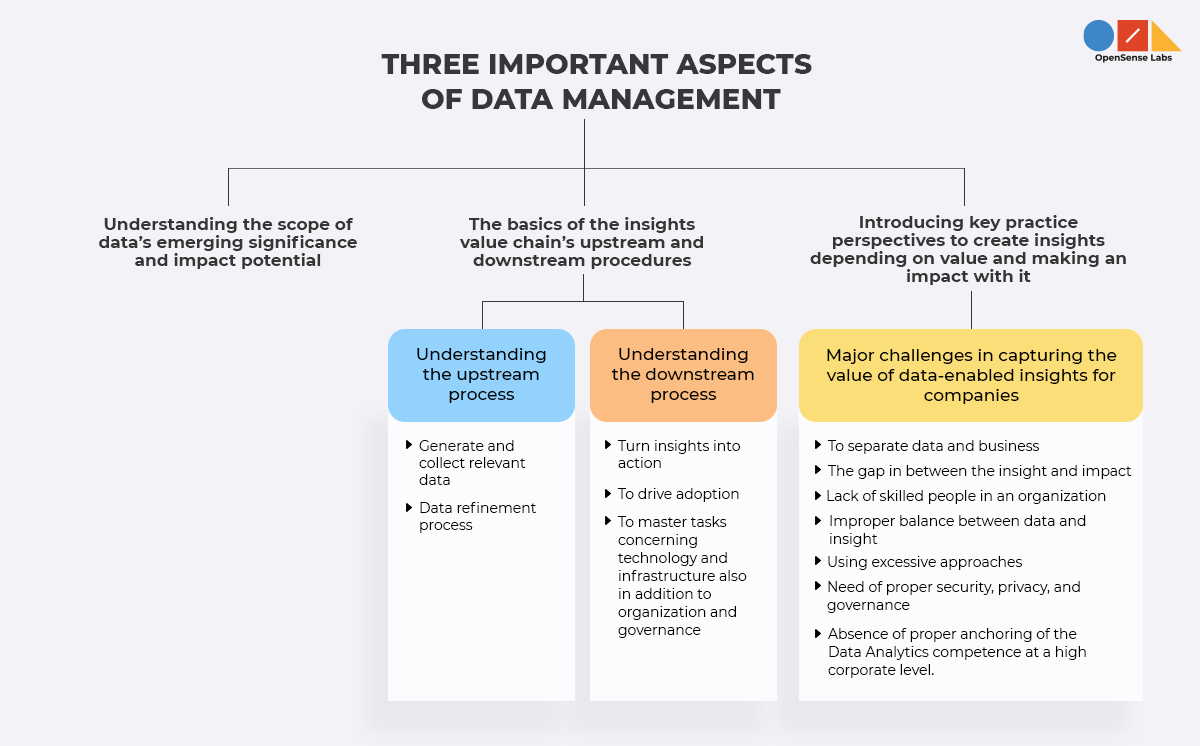
Understanding the scope of data’s emerging significance and impact potential
It is observed that a huge amount of data is mostly driven by the Internet of Things (IoT). Over the past five years, the availability of data has grown rapidly and is expected to grow also in the near future. There has been development of some new tools which can turn all these available data into insights and then finally into some action. Machine learning, a subset of Artificial Intelligence (AI), helps in leveraging algorithms to analyze huge amounts of data. You can find that Big Data and Advanced Analytics is making quick advancement and actively contributes in data management. Big data and analytics offer different kinds of value to different people within the company. Like for some professionals, it can be used as a strategic planning tool, a convenient way to recognize and focus on long-term business imperatives. For the other professionals, they deliver a quick, intuitive way to accelerate procedures and lead the path to change.
Also, visual analytics and discovery tools help in making the journey to data insight quick and easy. Instead of trying to take out meaning from a group of predefined reports or easy static dashboards, users are able to attain fast insights from data utilizing intuitive data visualization. Such modern visual analytics tools deliver easy-to-use data preparation features for a much better data wrangling.
We can see that data has not just increased in terms of volume but it has even obtained enormous richness and diversity. This era in which we are living is completely about digitalization. Data can be generated by everything including cameras and traffic sensors to heart rate monitors, allowing richer insights into human and “thing” behavior. Consequently, data can be considered as the new corporate asset class and companies are also achieving business impact with data very effortlessly. Companies can now digitize everything they do, and also digitizing customer interactions helps in providing proper information for marketing sales, and product development. Additionally, digitizing internal processes enables generating data which can be utilized to optimize operations and enhance productivity.
The basics of the insights value chain’s upstream and downstream procedures
The raw and most basic form of data is virtually valueless until you give it a voice by extracting valuable insights from it. Well, how can you make data speak? Everybody today speaks about Big Data, Advanced Analytics, Machine Learning and even AI. Since, people mostly prioritize these new technologies, more emphasis is given upon single technical components of the “insights value chain,” as we address it. But in reality, the entire series of components is required in capturing the complete value from convenient data.
1. Understanding the upstream process
Let us understand the insights value chain’s upstream processes.
Generate and collect relevant data
While watching where companies are up-to in the field of analytics, two trends came into notice. Firstly, algorithms appear to be commoditizing, for example, Google and Amazon are offering free access to their analytics libraries. Secondly, these companies are looking after their data by themselves, without giving access to outsiders, as they believe that the value can be found in the data. Collecting huge amounts of data is not only the domain of tech giants. Data’s value can be also identified even more in traditional sectors. For instance, to collect data on a bigger scale, joint ventures between telecoms and retailers are built. Such an improved data capture ability is not only suitable for B2C environments, but it is also essential for B2B.
You can see that as data generation and collection grow in volume, the data relevance becomes a priority. It is inconvenient to capture and save every part of the tera- and petabytes of data which gets generated every single second. Therefore, by defining particular requirements depending upon certain use cases, it will be helpful in capturing relevant data.
One another significant aspect of the generation and collection of data is data layering. Rather than throwing all the unconnected data into the analytics layer at a time, you can cautiously organize into various logical layers and then employ a logic by which you will be able to stack these layers that helps in generating meaningful data.
Data refinement process
After the company captures the relevant raw data, then the process of turning huge amounts of unrefined raw data into actionable insights needs to be started. So, the two-step process comprises enrichment and extraction.
- Step 1: To enrich data with supplementary information or domain knowledge
After the raw data gets collected, cleaned and combined, one of the most significant refinement steps get started i.e., transforming data and enriching it with supplementary and domain knowledge which is also a complex procedure.
In today’s world, both man and machine are considered as the optimal approach to data enrichment. But again, due to the advancement in the AI space, it might change one day. Therefore, before starting machine learning, there is a need to involve human experts who can be seen utilizing their expertise to explain their hypotheses. So, now a data scientist needs to translate, i.e., codify supplementary information or domain knowledge into variables. Specifically, it means to transform the existing data into new variables, which can be termed as feature engineering.
- Step 2: To extract insights using machine learning
The next step in making the raw data usable comprises the real math and number crunching which the data scientists are mostly excited for. But one might argue that there is no need for a human component as there are sophisticated algorithms which can find all the patterns. The reality is that the combination of human hypothesis-driven input and latest amazing patterns which the machines disclose is the successful combination. By creating new features, the machine can find better patterns and even help people to describe better and perfectly act on these patterns. This step is mainly about utilizing numerous methods to recognize these patterns. Basically, you can distinguish between descriptive analytics (what exactly took place in the past and why?), predictive analytics (what is going to happen in the future?), and prescriptive analytics (how can the future change?) In these steps, sophisticated methods are being used such as AI and machine learning.
2. Understanding the downstream process
The downstream process consists of nontechnical components. It comprises people, processes, and business understanding via a systematic approach that can operationalize the new data-driven insights through the help of an overall strategy and operating model leading towards better data insight action value.
Turn insights into action
After extracting significant insights from the models, the next essential step is turning these insights into action to create business impact. You need to have a proper understanding about the data analytics value chain. Also, it is stated that domain knowledge and business understanding play a significant role in being able to act on insights. In order to turn insights into action, it necessitates two things i.e., firstly, domain knowledge is essential in this case. Even though the mathematics of predictive maintenance for pumps on oil platforms may be similar to the telecom networks, the actions that are required to be taken later, will be different. Secondly, although once you get the clarity about what actions are required to be taken, success can still be based on how those actions are taken. Consequently, you will have to prioritize the processes and structures. For instance, in regards to the structure side, call centers may be reorganized, and save desks may be executed to provide support to the action of hiring specialized agents for customer retention. Apart from this organizational structuring, incentive schemes will have to be developed to motivate the success of these particular agents, since retention rates and not average handling times are in between their measures of success. Lastly, on the process side, automation may be introduced to activities like making pricing decisions upon online platforms.
To drive adoption
While talking about data and analytics, a commonly made mistake is to prioritize more on the new technical talent required. Even though it is certainly a significant and extremely scarce resource on the market, the real change needs to happen with the regular employees. These regular employees are required to increase their analytics quotient (AQ) and also become more data analytics. Also, the marketing, retention, and maintenance employees (among others) are required to understand which questions can be asked in regards to data and analytics, and they should be able to translate the answers, i.e., turn the insights into actions.
To master tasks concerning technology and infrastructure also in addition to organization and governance
The organizations can take action on the insights from advanced analytics and even create impact with the help of technology and infrastructure and organization and governance. Mostly, the organizations require the correct set of easy-to-use tools – e.g., dashboards or recommendation engines to allow personnel to extract the suitable insights and a working environment which facilitates the integration of those insights, e.g., governance which allows and manages the required change within the organization.
Introducing key practice perspectives to create insights depending on value and making an impact with it
After exploring the environment in which data is attaining significance and looking into the steps which consist of the up- and downstream processes of the insights value chain, let me take you through the major challenges in capturing the value of data-enabled insights for companies.
There are three structural challenges which prevent the clients from attaining maximum business impact from data.
- To separate data and business. In most of the companies, data science and business implementation are completely separate. Therefore, it results in lack of proper understanding from the business side of what is attainable and to the progress of data science solutions which the businesses do not require.
- The gap in between the insight and impact. In most of the cases, the essential step of moving from insight to insight-based value creation is never properly adopted. The company clients believe in the significance of analytics and have always conducted one or more proof of concepts (PoCs). These PoCs are mostly isolated from one another and never turn into successful use cases.
- Absence of proper anchoring of the Data Analytics competence at a high corporate level. It is observed that business impact from data-derived insights take place only when data analytics is implemented deep within and constantly all over the company. It needs a leader’s strong commitment and direction along with the authorization to drive this kind of insights-oriented transformation, and most of the companies are still to witness that level of company commitment.
Here are some of the common challenges that come along in the way of analytics excellence.
Lack of skilled people in an organization
After having well-structured data in place, the next thing you need is the right people with the right skill sets. But some organizations lack such skilled employees and it prevents the organization from proper utilization of the available data. Therefore, in situations like this, the talent management has to take the required initiatives in appointing the right talent in the organization.
Improper balance between data and insight
While trying to enhance the ROI on analytics, the companies face one of the common challenges i.e., the data itself. The real challenge for the employees is to extract the valuable insights from a huge volume of data. It is important for the team to know which data is utilized for which purpose, where they can exactly find it, and how they will be able to access it. It can be called data democratization.
In order to address this challenge, you will have to establish two main end goals i.e. company employees need to have a single source of truth for data, and the company needs to use data as a strategic, valuable asset which is not supposed to be misused.
Using excessive approaches
It is being proven that machine learning and other AI disciplines have come out as some of the best solutions for increasing profitable growth along with generating and scaling analytics. Due to the urgency of companies to adopt machine learning and AI, data and analytics leaders come across the challenge of trying excessive approaches i.e., using the latest technologies and methodologies without identifying the actual necessity of them in their organizations.
Need of proper security, privacy, and governance
It must be well known to you that security and privacy are the important factors which impact the success of the data program. While providing data access, you need to make sure that every access point and pipeline it touches is completely secure. Additionally, you should also be looking for the right data governance approach in the very early stage keeping the long-term goals and results in your mind, which will further enable you to execute and scale analytics much more easily. The needful approaches like ensuring your entire data stack, including warehouses, catalogs, and BI platforms should be compliant with data governance guidelines for your organization, industry and region as well.
Best practices
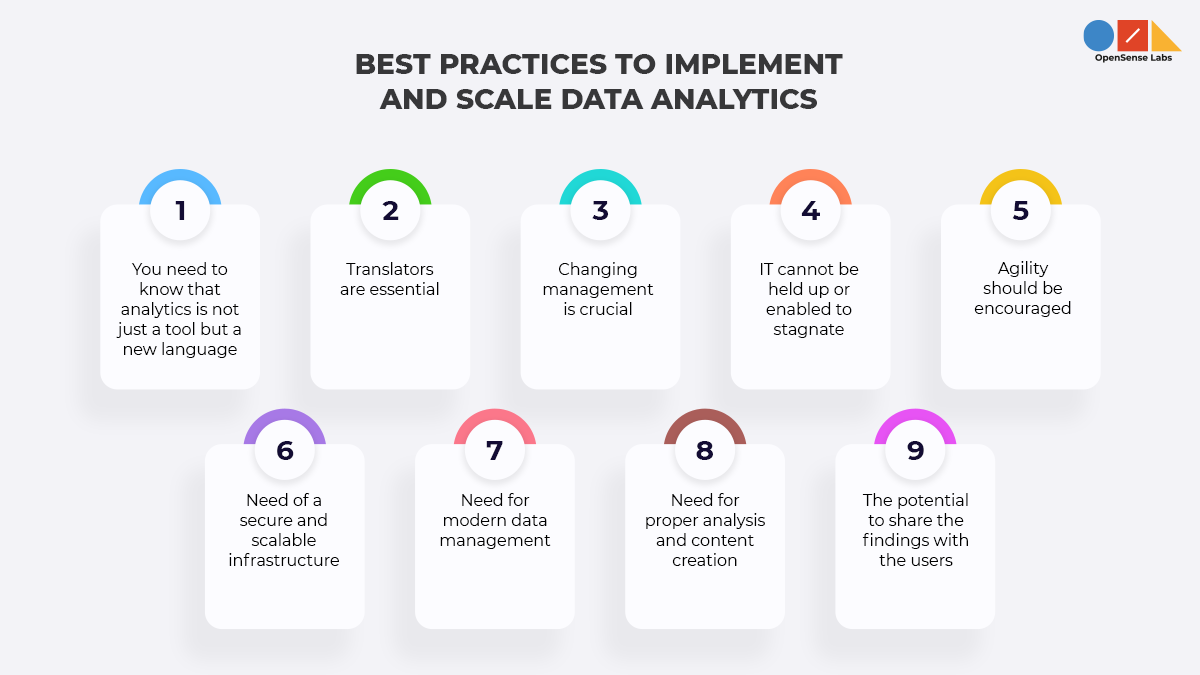
Let us now look into some of the best practices for executing and scaling Data Analytics.
You need to know that analytics is not just a tool but a new language
To act on analytics outputs, it needs the business to understand the implications of those outputs.
Translators are essential
You need to focus on hiring and training talent in order to serve as the interface between Data Analytics and business.
Changing management is crucial
The business users are supposed to trust analytics results, and they also should be able to act on them.
IT cannot be held up or enabled to stagnate
You are required to initially “bypass” corporate IT to start swiftly and show the concept early, yet you will have to finally involve IT timely and steadily for the purpose of scale.
Agility should be encouraged
The organization should be able to take risks in order to win big, without having the fear of failure. They need to embrace the experimental mindset and adopt the test-and-learn approach. The company’s cross-functional teams are necessary to keep pace with rapid test-and-learn cycles.
Need of a secure and scalable infrastructure
Since organizations deploy analytics over the organization to the maximum number of people, there is a need for better security and administration capacities. So, basically a secure, scalable infrastructure is required which can provide support to self-service data analysis.
Need for modern data management
The modern business intelligence platforms should be able to offer support in order to extract, transform, load (ETL) processes and data preparation in a self-service manner. They should be offering in-memory or other data storage and optimization mechanisms which are integrated straight away into the platform. Additionally, metadata management and governance are also main capabilities that help in advancing analytics.
Need for proper analysis and content creation
An important fact is that an interactive visual exploration is a very powerful procedure which helps in attaining much better insights from data. Therefore, the data professionals are today finding ways to create content, calculations, and algorithms, and analytic content that helps in leveraging more advanced analytics beyond basic aggregations.
The potential to share the findings with the users
In order to make the insights reachable to more users, companies should be able to swiftly embed analytic content in a dashboard, app, web app and portal. They may also want to publish analytic content to dashboards and reports, print it, distribute it, schedule it, and also have alerts which generate the content. Also, the collaboration and the social capabilities enable users to share the finding, examine and evaluate the content.
Lessons for business leaders
Now, I will take you through a section where we will find out how the company leaders can smartly harness the data in a manner which generates insights that can further turn into value. So, according to the survey conducted by Harvard Business Review, it is observed that one-third of the 311 survey respondents have considered themselves to be data-to-value leaders (“leaders”), since their companies are very efficient in harnessing data in order to create new business value.
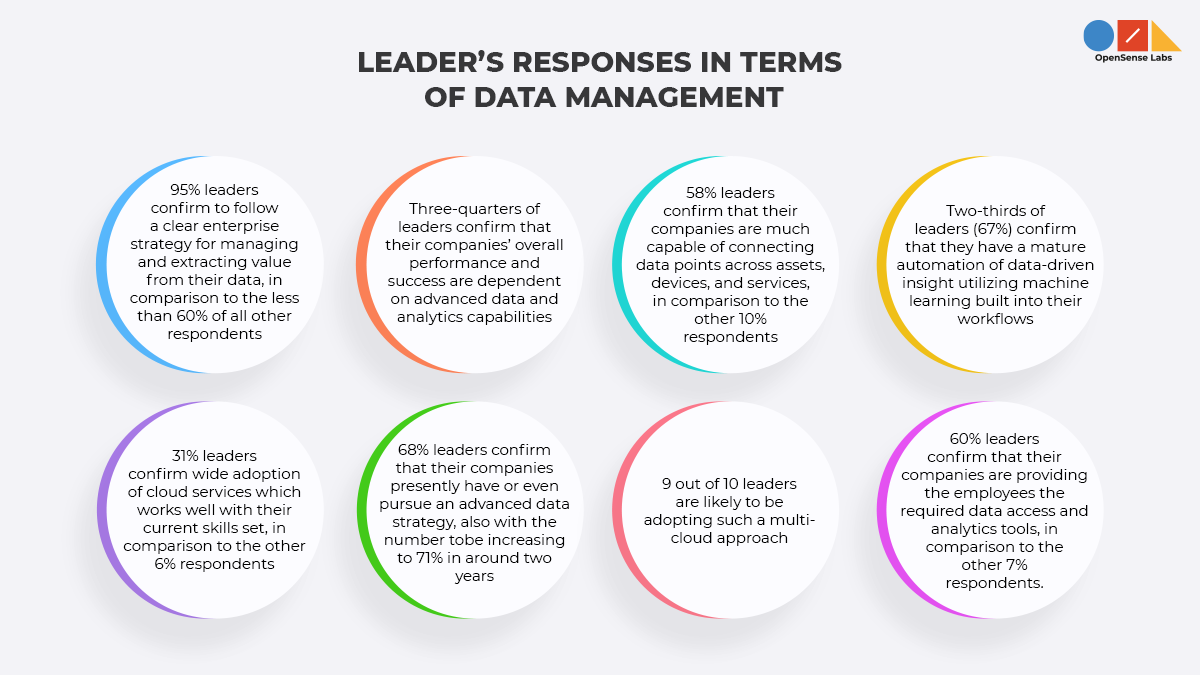
So, basically more than 95% of these leaders hold a very clear enterprise strategy for properly managing and extracting value from their data, and for collecting and incorporating external sources of data, in comparison to the less than 60% of all other respondents. Therefore, such leaders are intelligent, smart and up to date.
During the survey, when the leaders were asked about the significance of three advanced data and analytics capabilities in order to connect data points over a variety of assets, devices, and services; to access and analyze data in real time; and to automate data-driven insights, they tried differentiating themselves from the other survey respondents. Almost three-quarters of leaders shared that their companies’ overall performance and success are majorly linked to each of these capabilities, on the other hand, the rest of the respondents were much less likely to say so. Similarly, a large number of leaders confirms having mature capabilities in each of these areas. 58% share that their companies are much capable of connecting data points across assets, devices, and services, in comparison to the other 10% respondents. The same 58% also confirms that their companies have mature, real-time data access and analytics capabilities in comparison to the rest of the 6% respondents. Also, around two-thirds of leaders (67%) express that they have a mature automation of data-driven insight utilizing machine learning built into their workflows, while the 5% of the left out respondents say so.
One more thing that came out in notice was how the leaders prioritize the cloud services adoption. 31% leaders confirm wide adoption of cloud services which works well with their current skills set, in comparison to the other 6% respondents. In the companies, multi-cloud strategies are gaining importance. Around 68% share that their companies presently have or even pursue such a strategy, also with the number to be increasing to 71% in around two years. With nine out of 10 are likely to be adopting such a multi-cloud approach. This multi-cloud approach offers much greater operational efficiency that is mentioned as a top-three driver by 42% of all respondents, performance optimization (35%), cost optimization (33%), increased agility (31%), risk mitigation (30%), and access to best in-breed solutions (24%).
One more important thing the leaders adopt is the need of utilization of data and analytics in every part of the company, where it can offer value, which is basically everywhere. So, in order to do that they have to replumb the data supply chain, starting from ingestion to disposition. Around 60% of the leaders stated that their companies are providing the employees the required data access and analytics tools, in comparison to the other 7% respondents.
After this survey, it is proved that the leaders are taking proper initiatives and steps in valuing data and turning them into business value.
Use cases
Now, let us look into some of the organizations which have collected their disparate data together and turned it into a huge success.
Telefónica bringing suitable data together to increase satisfaction and drive growth
Telefónica Spain has been investing in cutting-edge technologies for a long time. With a count of million customers and more than 17 million mobile customers, the company sticks to its commitment towards innovation to know its customers on a better perspective, personalize services, provide data control and security, and also maintain industry leadership. Telefónica thought of taking advantage of its huge volume of customer data by combining it under a single data warehouse and also applying real-time analytics to enhance operations and the customer experience.
In order to connect to the customers touch points over marketing, service and sales automation and make them even smarter, this company selected Oracle, to help in storing more than three petabytes of data which is constantly analyzed. While previously it took 20 days to describe a target audience for a CRM campaign, now the company is able to market to a particular audience in real time. The solution is also able to power the company’s television recommendation engine, enhancing customer satisfaction and driving business growth.
More on handling customer data the right way:
- Ultimate guide to customer data platform (CDP)
- How to know if a CDP is right for you
- CDP vs CRM
- How to choose the right customer data platform
- How to successfully implement CDP
- How customer data platform supports personalisation
- The case for customer data privacy when using CDP
mStart consolidates data to improve retail experience and efficiency
mStart is a Croatian provider of business and technological IT solutions for the agriculture, industrial, and retail sectors which is continuously attempting to optimize procedures and enhance service delivery. It was seeking for a complete detailed insight into the shopping behaviors and requirements of global retail customers, and was also looking to optimize its supply chain procedures. So, Oracle helped mStart in establishing a data lake which offers almost real time, detailed insight to the activities of more than 2,000 stores. The solution allows retail outlets to smartly make same-day amendments to prices and promotions, to boost revenue and optimize inventory management. mStart, which is combined with Oracle Retail Demand Forecasting and Oracle Retail Replenishment Optimization, is able to improve its supply chain procedures, and anticipates transportation cost savings of 10 percent within a period of three years.
For better understanding you can go through this Q & A Session between Mukesh Jain, Chief Technology & Innovation Officer, Vice President & Head – Insights and Data Technology, Capgemini, and Noah Schwartz, GM, Product and Engineering, AWS Data Exchange in regards to utilizing data to drive business growth and value.
Understanding data strategies to enhance business value
Forbes has come out with three strategies which are required to be the base of any digital transformation strategy.
- You need to build a single view of your customer. Suitable data comprises purchase information, communication preferences, shopping behavior and social media activities. It necessitates connecting structured data (like addresses, household composition, socio-economic bracket, etc.) with unstructured data (like the free-form text of a Twitter feed). More on improving consumer behaviour with a perfect data driven strategy here.
- You need to use the location data to add precision and context. In this highly advanced world, there is no need to know about your customers. All you need to know is their place to provide a real hyper-personalized and responsive experience.
- You need to create suitable communications at the right time on the right channel. Since data displays significant details about customers, it's really necessary to be able to engage them via different points of conversation.
The next thing which you should know is that the data strategy has to properly align with the business outcomes. But before that you should essentially know what are data strategies? And, how do you develop a data strategy? Only then you can have an added advantage by planning a successful data strategy. So, many of the business outcomes are classified under these below categories.
Empowering your employees
Consider allowing your employees with real-time knowledge of customers, devices, machines to efficiently collaborate in order to meet customer or business requirements with agility.
Engaging with customers
You can deliver a personalized, rich and connected experience to your customers. By harnessing the power of data and insights, you can drive loyalty along each step of the customer journey.
Optimizing operations
You need to increase the flow of information over your complete business operation. Keeping your business procedures synchronized and making each interaction meaningful via a data driven approach is required.
Transforming your products and development lifecycle
You need to collect telemetry data about your services and offerings. You can further utilize the data to prioritize a release or create a new feature, and evaluate efficacy and adoption constantly.
Finally, now let’s look at the strategies that can drive your business value at scale.
Challenging the basic assumption that restricts the freedom to experiment
One of the most important challenges the companies face is the lack of access to centralized data to get any value out of it. Due to this difficulty, the companies are unable to get engaged in data-driven experimentation for gaining better business outcomes.
Since, data keeps on changing, and you would want it to change, along with that you wish to have new customers and markets, therefore, you will need to build a structure which is reactive to change and also adaptable. In order to move from having data to utilize it for a basis for products, personalization and seamless customer experience, you need freedom to experiment. Therefore, monolithic data architecture helps in making this task monumental, by extending the gap between theory and action. There is also a tooling aspect to it which can dictate the cycle time it takes in making decisions.
Organizations to prioritize an agile approach to data management
Organizations need to adopt an agile approach to data, also bring it closer to parts of the business wherever it’s suitable. It can be attained by applying two main principles i.e. domain-oriented data and data as a product. Domain-oriented ownership and distribution helps in breaking down data architecture around single functions, at the same time maintaining an extensive connectivity and integrity. Then utilizing data as a product instead of a resource becomes easy to consume and utilize. These practices are the fundamentals of a data architecture that is well designed for a resilient, quick-acting, digital business: Data Mesh.
Data Mesh looks at the root cause of the inability to use data at scale, and tries to address it.
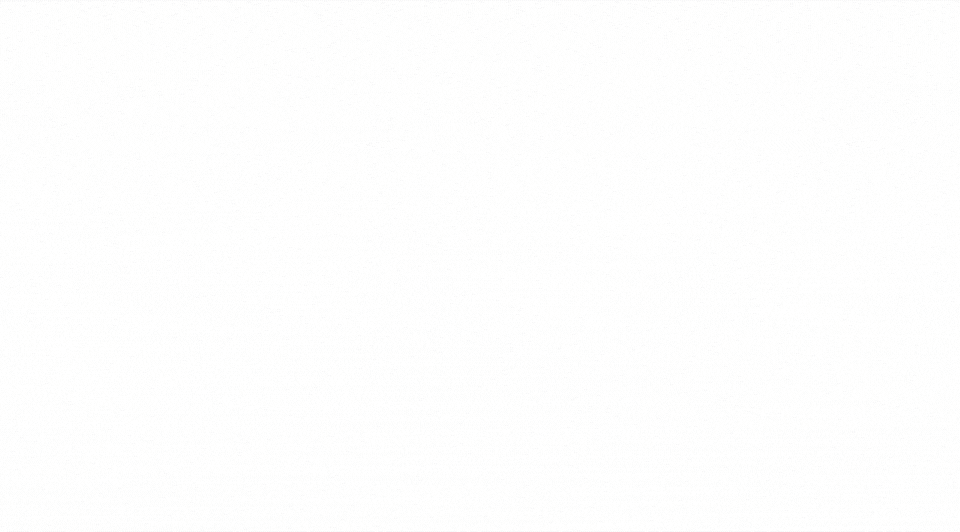
Designing a modern platform architecture
It is observed that self-serve platforms help in positioning data that can be put into instant use, instead of keeping it stored and being accessed before going through other procedures. Moreover, modern platform architectures enable us to get closer to data and get the insights at the earliest, also keeping us away from the chaos that is experienced with data engineering. These architectures prove to be perfect enablers for the companies which position their respective teams to get access to standardized data capabilities which can be further assembled to create various products. While developing a data platform, companies will not always start from scratch. So, the current cloud technologies can act in the form of a utility layer, enabling the storage and streaming capabilities and standards over which more mature layers of the platform can be built in order to support communications with distributed architecture and also decentralized teams.
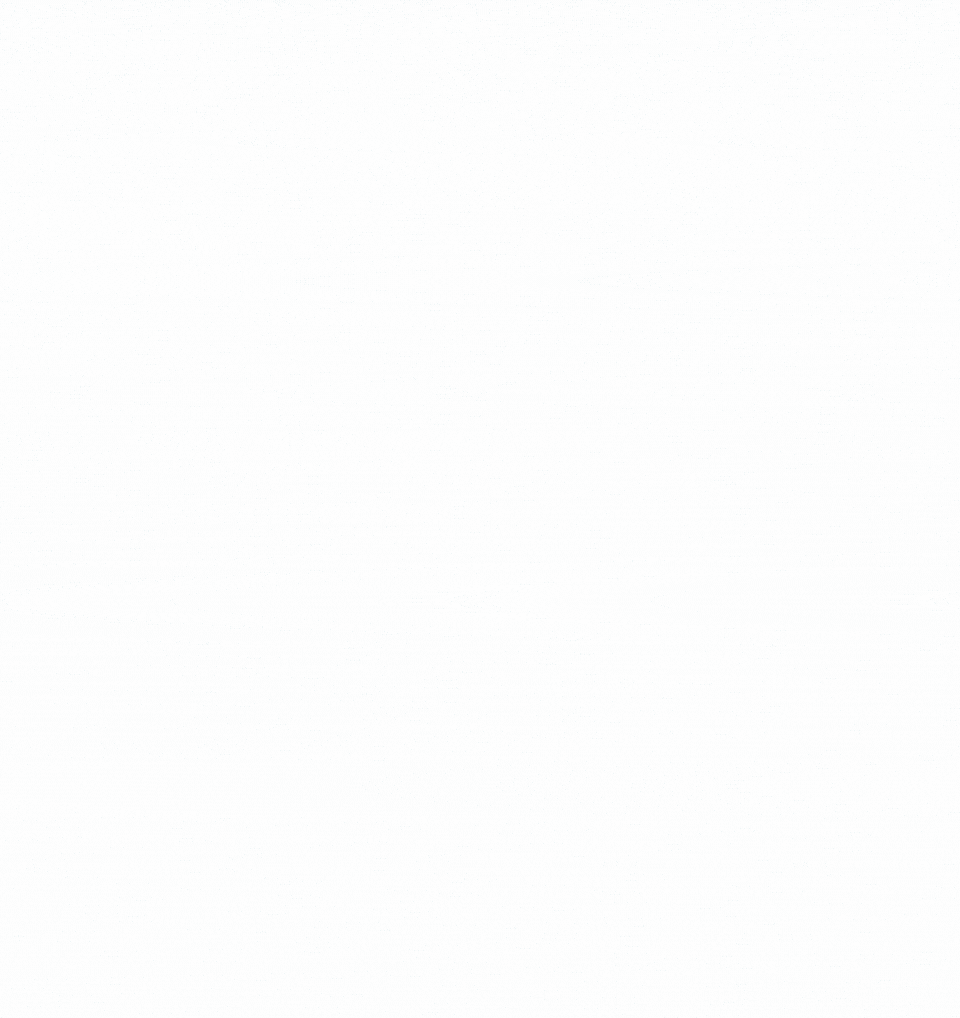
Making your people believe upon the strategies
Even though data architecture can be complicated, in order to become more data-driven, you will have to prioritize people and culture rather than focusing on technology or engineering. Prasanna Pendse, Head of Technology, India, at Thoughtworks says, “The truth is, people look at data and particularly data governance and their general reaction is to groan. It’s seen as boring, something they have to manage, a burden. Leaders may believe in it, but they can’t get buy-in from their teams. These perceptions have to change, so people are interested, want to use and consume the data, and are excited about the possibilities.” In order to help this situation, the first and foremost thing you need is a clear and extensive data strategy. So, what is the value of a data strategy? It has to come via a culture of evangelization of what exactly data means and why it is important for the company, like it is for creating more insights, developing more products, regulatory reasons and process control. No matter, even if it comes from C-level but is needed to be embraced by all the executives in the company. Also by describing the strategic purpose of data, it helps in deciding which data and related solutions to be prioritized.
There is no harm in starting small and being ever ready to grasp the learnings. You need to have feedback cycles which will help in identifying what really works for you and what exactly needs to be adjusted. The authority shall be given to the teams to develop things and to make the required changes. By finding the right people, you can provide them the necessary time and space for making the changes.
Handling the data talent shortage
In companies we sometimes find that there is sufficient data talent but people don’t realize it. Like, some people might have interest in data but they are restricted from communicating with systems or even working with developers since tasks like this do not come under their formal role. In this situation, “Data Mash” concept can really be helpful. It is about integrating responsibilities more upon the domain teams, allowing people to use sandbox environments, providing them complete access. You just need to allow people to get more experienced with data systems, reduce their fear and not be too strict with them. It is also preferable to reskill or train even the existing talent since they can offer more return on investment rather than hiring new data specialists.
The closure
From this article, I hope it is clear to you that companies are striving to become completely data-driven in order to gain better business results and success. They are using several platforms to analyze huge volumes of data and utilize them to develop competitive advantages. At times it can be a challenging task but the company leaders are well determined to take the needful actions for providing excellent services to their customers and to enhance their business efficiency.
Subscribe
Related Blogs
Exploring Drupal's Single Directory Components: A Game-Changer for Developers
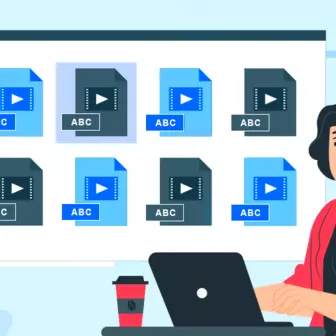
Web development thrives on efficiency and organisation, and Drupal, our favourite CMS, is here to amp that up with its…
7 Quick Steps to Create API Documentation Using Postman
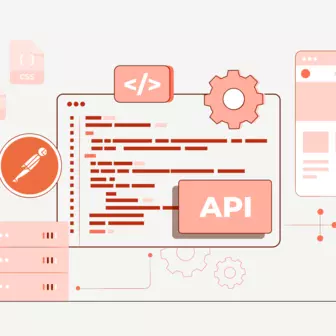
If you work with API , you are likely already familiar with Postman, the beloved REST Client trusted by countless…
What is Product Engineering Life Cycle?
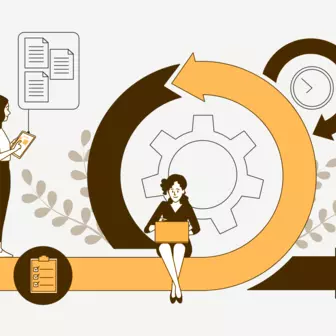
Imagine constructing a house without a blueprint or a set of plans. It will be challenging to estimate the cost and labor…