A black spot on a white sheet of paper can be found with a quick glance. What if you have to search for a black dot with certain radius among the cluster of dots on a large sheet of white paper. Such is the need of the hour where you have to intelligently search for a piece of information from a cornucopia of data in your system. Cognitive search is revolutionizing the process of retrieving the files.
There is a diminishing trend of manually searching for a document stored somewhere in your system. Large enterprises are the ones who are showing their dire inclination towards this disrupting technology.
Before we move on to how large organizations are looking to extract the merits of cognitive search, let’s understand what it is.
What is Cognitive Search anyway?
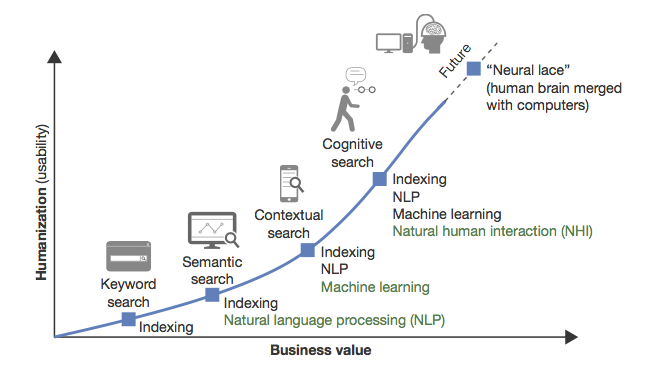
Forrester, research and advisory firm, defined cognitive search and knowledge discovery as “the new generation of enterprise search solutions that employ Artificial Intelligence (AI) technologies such as natural language processing (NLP) and machine learning to ingest, understand, organize, and query digital content from multiple data sources”.
That is the best definition one can give to describe a cognitive information system. In short, it can extract the most relevant piece of information from large sets of data in their work context.
Platforms enabled with cognitive computing abilities can interact with the users in a natural manner. With experience, they can learn user preferences and behavioral patterns. This helps them establish links between related data from both internal and external sources.
How Beneficial is Cognitive Search?
So now we have an understanding of what it is and how it works. How can it turn out to be a great asset?
Tapping into large sets of data sources
- To fetch the best piece of data out of voluminous sources of data can seem tiring. Cognitive search can work wonders in extracting the most valuable piece of information from large sets of intricate and varied data sources.
- Whether it is internal or external, it peeks inside everything that is available in your entire enterprise. It also touches searches through structured and unstructured data and lends deep and insightful search capabilities to your organization. This helps in making better decisions in the business.
Providing relevant knowledge
- It comes packed with a lot of functionalities that leads us to find meaningful and relevant information. Doing a search across the enterprise data may seem daunting, but it does that with ease.
- Using NLP, it can gauge and get to know the scheme of things vis-à-vis text content like email, blog, report, research work, and document and also media content like meeting videos and its audio recordings.
- Once it is done with the understanding part, machine learning algorithms help it do deeper research and come up with insightful information. Company dictionaries and ontologies help with understanding the terminologies and their relationships.
Enhancing search results
Machine learning algorithms help in providing better search results.
- To help digital marketers predict if the advertisements designed by them is going to work or not, a supervised learning algorithm called Classification By Example can help. For instance, it can help them judge how people reacted to particular ad campaigns in the past to help them come up with something better this time around.
- Marketers can ascertain a particular group of people and target them for their upcoming marketing campaigns. Clustering, an unsupervised learning algorithm, helps them in the process.
- To understand the relationship between input and output variables and do the prediction, regression algorithm comes handy. For instance, it can be used to build applications that determine the road traffic based on the present weather situations. Also, based on various economic factors, it can help predict stock prices.
- Similarity algorithm can help you appoint an expert team for a business project based on their skills and competency levels in the previous projects.
- Personalized recommendation based on the interests of the users can be done using Recommendation algorithm. Based on the previous history and usage patterns, it can recommend content which a user would most likely want to consume.
How did Cognitive Search come into existence?
It is a valid question. One would ponder whether this was developed in a short span of time or did it involve some amazing technologies behind the scenes. It was, of course, a very long roadmap which had to be traveled to reach this tech marvel.
By now, you have already noticed that, as the definition mentions it, machine learning and artificial intelligence are the frontrunners in leading up to this masterpiece.
Almost all the search methods that exist today are some way or the other related to Google. Leverage Marketing came up with an interesting study. When Google first created a search engine in 1996, there were already several others in place. But Google’s search was different. While other search engines delivered search results only if they could find an exact keyword in the search box, Google had a different algorithm.
Google gave a value to certain keywords. So, keyword frequency determined the search results which led to irrelevancy in terms of content that it showed on top. So, in the 2000s, Google devised several improved search techniques. It, finally, incorporated machine learning into their search engine in 2015. That means Google would not just read what you have written in the search box but interpret what we really mean when we type that.
By developing its cognitive learning search method, Google’s search algorithm could understand keywords and provide rankings, past search results, browser history, user location and other such parameters. This was their major leaning towards artificial intelligence.
This is how Google standardized the internal search. Office network developers contemplated developing search methods for their business needs. Their pursuit of search method development was based on Google cognitive search and machine learning techniques. That is how cognitive search came into existence and did a splendid job in improving the search experience.
Challenges that lie ahead...
Three-pronged approaches to look at the challenges that it might encounter and how to tackle them:
- Expertise: Shortage of personnel required to develop and maintain this budding technology can be one of the main challenges that have to be overcome.
- AI implementation:
- Supervised machine learning helps in recognizing user patterns over time. Providing sufficient labeled training datasets from which these systems can learn is a huge challenge.
- Unsupervised machine learning identifies existing user patterns. Systems with this capability face a major hurdle. Sufficient data with intervention for proper guidance and interpretation to train the system is a challenge.
- Goal formulation: There has to be clear goals and outcomes formulated. For instance, in reinforcement learning, systems perform several attempts and learn from the outcome of the trials to take better decisions. The biggest task is to provide clear-cut goals and enough practice to the systems in a challenging environment.
How can Cognitive Search improve Enterprise search?
Large enterprises are having this question as this will make life easier. We have seen that relevancy, meaningfulness, and completeness are required to get the better search results. But they should also have the enterprise qualities.
- Understanding data: It should understand any data that an enterprise would fling at it. It should browse through the plenitude of data sources, understand both structured and unstructured data to come up with better enterprise search results.
- Scalability: It should be able to scale with the ever-increasing density of enterprise data. Large enterprises have hundreds and thousands of applications with several bytes of data stored in the cloud or on-premises. Cognitive search solutions should deliver better quality search results.
- Manual fine-tuning: It uses natural language processing and machine learning algorithms to understand data, do the prediction of user’s search patterns, improve the relevance of search results and automatically tune them over a period of time. Cognitive search solutions should provide tools for administrators to manually tune search results. After all, AI is not perfect.
- Building search applications: It should help developers to develop search applications. Instead of incorporating simple text box search methods, business enterprises should be able to build an application that works like virtual digital assistants such as Google Now and Siri.
Use Cases
Sinequa, which provides cognitive search and analytics platform for over 2000 organizations, has a great cognitive computing solution. As a matter of fact, it is recognized as the leader in the Gartner 2017 Magic Quadrant for Insight Engines and the Forrester Wave™: Cognitive Search and Knowledge Discovery Solutions Q2 2017.
Sinequa partnered with content platform firm Box to enhance cross-platform enterprise search and analytics.
- The Partnership between Sinequa and Box helped in leveraging the aggregation of human-generated data in an enterprise, exploring information, and apply them in a business decision makings. This integration not only lets customers mine their Box content but also allows to search across other organizations’ repositories. This increases the value of the information searched by linking them with related content across different sources which was previously affected by standalone silos.
- Sinequa offers more than 150 connectors to its various data sources. And with this partnership, the relevancy of search results for the enterprise data corpus has only got improved. Individuals can do the enterprise search across platforms for contextually relevant search results across platforms from a single interface. Hence this shows that their partnership has promoted the enterprise cloud-first philosophy that is becoming the norm of the industries.
- From the angle of information management, the partnership is significant with both the companies having a huge presence among the large enterprise clients. Box provides the user-friendly cloud file sharing and sync functionalities. It decided to embed enterprise governance features for the content by building tools for reports, access controls, workflow, securities policies etc. Partnering Sinequa further strengthened the voluminous knowledge base content of Box by allowing it to maintain control settings on data while the content is being searched and assessed on Sinequa.
- Native security and permissions settings of connected repositories are preserved to a great extent with this integration. Users can search the Box environment without bothering the native control settings on the respective platforms where the information is located. That means Sinequa search interface allows users to endlessly search for the content and the granular security and permissions settings of the Box remain intact.
- 360-degree view of the customer is attained through this partnership. Sinequa’s natural language processing and machine capabilities, powered by Apache Spark, and its more than 150 connectors to content sources let it form compound results of the enterprise search for detailed understanding by humans. If a user is searching for a specific subject in Box, he or she can view most contextually relevant results from email, Salesforce, on-premise file shares and other such sources. This mitigates the time and efforts required to find the right actionable insight. Thus, this improves yields from certain initiatives like gaining a 360-degree view of the customer.
Hewlett Packard Enterprise (HPE), multinational enterprise information technology company, has its own cognitive computing solution. Delivering natural language based systems to meet the ever-increasing needs of users, providing answers that are precise, relevant and trustworthy is important. HPE IDOL Natural Language Question Answering is one such solution that comes with natural language processing enables features for large enterprises.
- Accurate response: IDOL Answer Bank feature helps in providing accurate and curate responses to the predefined reference questions. For instance, It can be programmed to give you instructions on configuring a smartphone.
- Fact-based answers: IDOL Fact Bank feature helps in providing answers based on proper facts. For instance, it can give the stock price details through structured data sources. Or it can provide company's annual report through unstructured data sources.
- Text-based overview: IDOL Passage Extract feature helps in giving you an overview. For instance, you can see the latest financial services and their rules and regulations or the news events.
- Assessment questions and data sources: IDOL Answer Server feature assesses the questions and various content sources to provide the best possible answer.
Cognitive Search solution providers
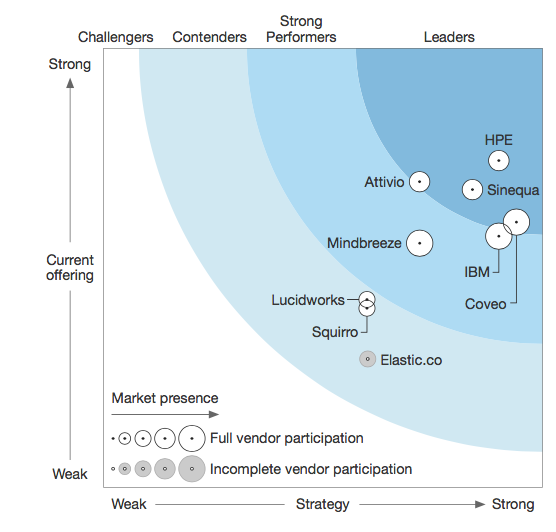
Forrester, in their research study for Cognitive Search and Knowledge Discovery Solutions, compiled a list of high performing vendor solutions.
- HPE IDOL: This solution is built to analyze everything that is searched using it. With HPE’s intentions apparently not restricted to unstructured text, its cognitive computing platform also does a deep analysis of speech, images, and video. It includes capabilities like gauging a question and optimally answering that can help developers in developing chatbots or virtual conversational assistants.
- Coveo: Its major focus lies in contextual and relevant search results. It uses advanced analytics and machine learning algorithms to return the most contextual results for the queries made by the user. It has also integrated with Salesforce using its cloud-based model.
- Sinequa: It gave importance to natural language processing for the better understanding of search queries and relevance of content discovery. Moreover, incorporating Apache spark, its analytics platform has got a further boost.
- Attivio: It is suitable for most complex search applications. It offers knowledge management, anti-money laundering, customer 360, and other such features. Developers can use the structured query language to search the index.
- IBM: It has leveraged the utilities of Watson Explorer by incorporating it in IBM’s Watson Developer Cloud. Watson explorer can be deployed in the cloud or on-premises. It is very helpful for customer 360 search applications, enterprise search, and claims processing.
- Lucidworks: Their solution called Fusion has fantastic enterprise search features, 40 prebuilt connectors to applications like Salesforce and Slack, better administration tool, and out-of-the-box machine learning algorithms to come up with better knowledge discovery.
Summary
Cognitive search has emerged as the default standard for enterprise search. By analyzing a search query, using its AI capabilities, to give the most relevant and contextual output, it has led to a volte-face in the thinking of large enterprises.
Using internal and external content sources to provide the most relevant knowledge and enhance search results, it has been a huge helping aid in the smart cross-platform search.
Google’s search engine integrated it in their algorithms to understand user’s behavioral patterns and show results. This is how cognitive search came into existence in the enterprise world.
It has to break through the straitjackets of few challenges to come out as an improved technology in the coming years.
With a deep understanding of data, scalability with challenges and manual tuning by administrators etc. it can improve enterprise search.
Leaders in cognitive search solution providers like Sinequa, HPE, Attivio among others have amazing platforms where customers can reap the benefits.
We love cognitive search. Contact us at [email protected] to implement this in your property.
Subscribe
Related Blogs
Why should you prioritize lean digital in your company?
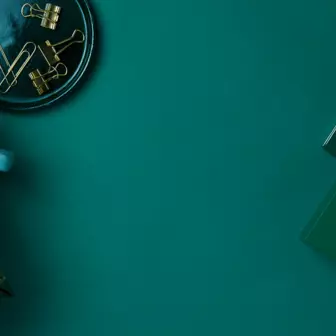
We are living in an era where the change and innovation rate is just so high. If you want your organization to reach new…
How to measure your open source program’s success?
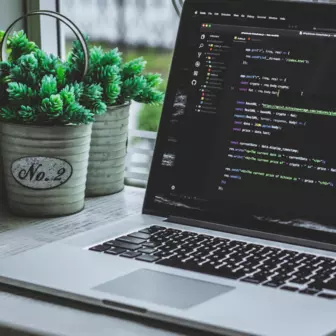
Along with active participation, it is very important to look after the ROI of open-source projects, programs, and…
Understanding the significance of participating in open-source communities
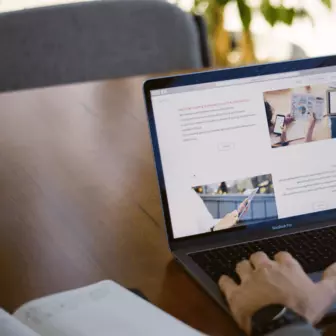
Do you think contributing to the open source community can be difficult? I don’t think so. Do you have to be employed by a…